The rapid advancement of technology has paved the way for more innovative tools in the field of data annotation and computer vision. One of which is the Segment Anything Model (SAM) developed by Meta AI Research. In this easy-to-understand guide, we're going to cover a few important topics.
First, we'll explain what 'segmentation' is all about. Then, we'll tell you about SAM, a really cool model that's changing the game. And finally, we'll show you how SAM can make your annotation work in CVAT faster and easier.
What is image segmentation?
Imagine you have a big, complex jigsaw puzzle with lots of pieces that together form a picture. Now, suppose you want your computer to understand this picture, not just as one big image, but to know what each piece of the puzzle represents.
Image segmentation in data annotation is like helping the computer to put this jigsaw puzzle together. It's a process where we tell the computer to separate or "segment" different parts of an image into smaller sections, each representing something specific.
For example, let's say you have a picture of a park. In this picture, there are trees, people, dogs, a bench, and the sky. With image segmentation, you can tell the computer to identify and separate all these different elements. The computer outlines trees, people, dogs, the bench, and even the expanse of sky. It recognizes each pixel as part of the respective object, attributing each tiny point of color to its designated element.
After this process, the computer will not just see a picture of a park, but it will understand the individual components that make up the image. It can tell you where the trees are, where the people and dogs are, and where the sky and bench are.
That's what image segmentation is all about – helping computers understand images at a much deeper level by teaching them to recognize and differentiate between different parts of an image.
In the world of computer vision, data segmentation is especially tough because it requires a ton patience and attention to details from data annotators. The job is hard because images for annotation are never simple and straightforward: there are all kind of shapes, things overlapping each other, not always clear where one object ends and another begins. To sum up – you need a lot of expertise and practice to do image segmentation fast and precisely.
What is Segment Anything Model (SAM)?
The Segment Anything Model, or SAM for short is a tool designed to help with this tough job of image segmentation. What makes SAM special is its ability to work with any object in an image not just specific ones.
How did SAM become so good at this? Well, it learned from a huge library of images and examples. SAM's dataset, called SA-1B, is the biggest of its kind. It has over 1 billion examples on 11 million images that respect privacy rules. This data set, supported with proper algorithms, made SAM a groundbreaking model in the area of image annotation.
If you are interested in more technicalities about how SAM works, take a look into official papers here: Segment Anything Paper.
And we are coming back to simple explanation In simpler words. SAM is a game-changer for people who work with data. Just one click and SAM spots and separates all different objects in a picture. It's a big relief because doing this manually used to be a real time-eater.
As the saying goes, "a picture is worth a thousand words." To truly understand the speed and efficiency that SAM brings to image segmentation, let's show you rather than tell you:
SAM Model in CVAT Cloud
When we pair SAM with a tool like CVAT, it's like a breeze to segment images. That's because CVAT is like a bridge that connects SAM with the images you're working on. This way, you can separate objects in your images with a simple click. It's as easy as that!
First went self-hosted solution, now SAM in CVAT cloud is available for everyone.
The process is simple:
1. Create an account or log into the existing one.
2. Create task and upload dataset.
3. Annotate!
The SAM model is available from the AI Tools > Interactors.
To try it out, click here!
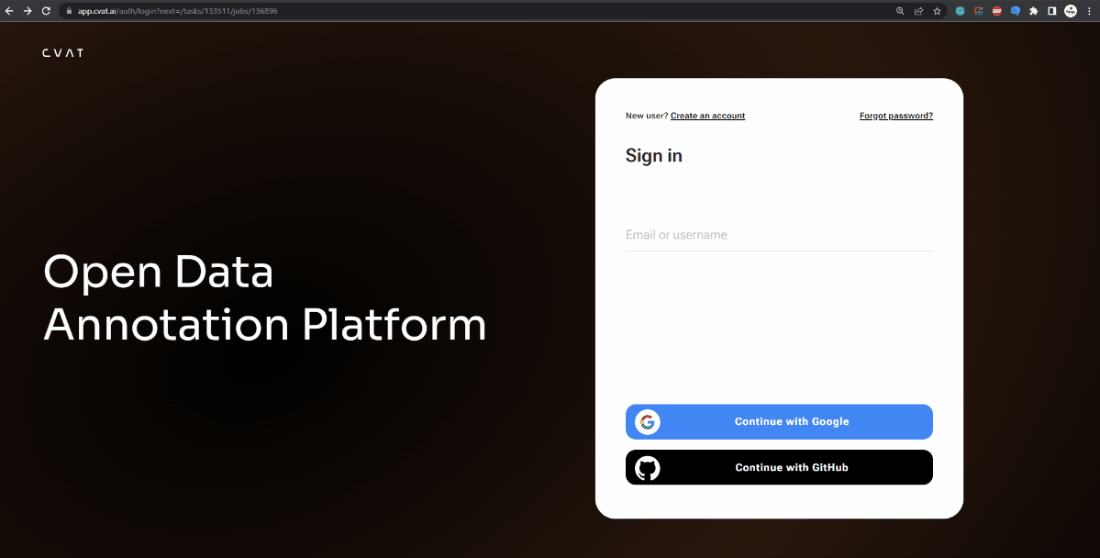
Stay tuned and follow the news here: